The last time you were planning a major purchase – a new laptop maybe, or a holiday at a hotel – did you check out online reviews of what other people thought before you parted with your money?
Then maybe, post-purchase or holiday, you left your own opinions online to encourage or warn other would-be consumers.
Online reviews form a trove of information, and Prof Barry Smyth at University College Dublin is digging into them for opinions and sentiments – or rather the software his lab develops is doing the digging – in order to build new recommender systems.
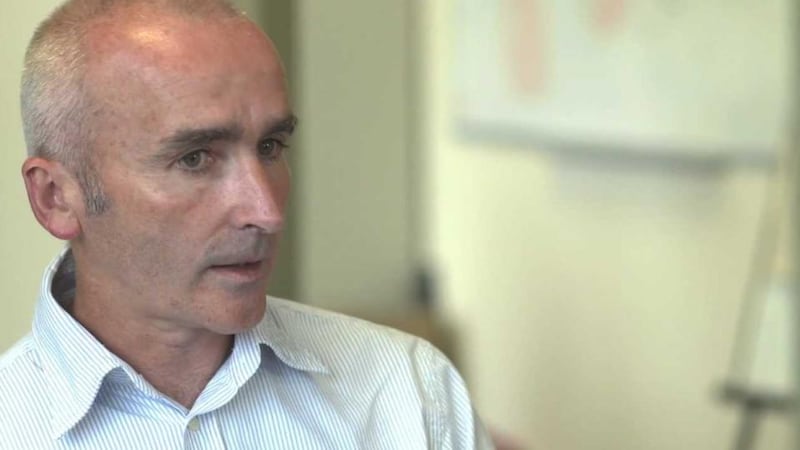
“People are influenced by reviews, and very often people use online stores to do their research on a product,” says Smyth, a director at The Insight Centre for Data Analytics. “And I’d never dream of booking a hotel without checking out reviews online.”
The recent case of a hotel in the United Kingdom reportedly fining (and later refunding) a guest for leaving a negative online review highlights how seriously reviews are taken, says Smyth, who is developing software to analyse what he sees as a useful vein of information.
Of course, opinion mining and sentiment analysis are not new.
The field has been fuelled by the growth of social media providing plenty of content, and algorithms can now analyse what people are talking about online, classify whether the tone is generally good or bad and even summarise content automatically.
“That’s all part and parcel of what people are doing in the mining space,” says Smyth, who holds the digital chair in computer science at UCD.
“But I’m interested in seeing how we can mine online reviews to help improve recommender technology.”
Recommend this
The idea is to use software to recommend products or services based on user experiences rather than relying on star ratings, purchasing information and providing the ‘other people who bought this product also bought . . .’ suggestions, he says.
When reviewing, users may mention features that are not listed in catalogues – perhaps they were struck by the quality of a breakfast in a hotel, or the battery life of a laptop – and they might even say whether or not they like them.
“This is where the richness comes in. Using this information you can get a whole new generation of recommendations that are more insightful, because they are based on real user experiences, not just on catalogue information.”
Lifts and carpets
His lab recently carried out a study of more than 148,000 online reviews in September 2013 for about 1,700 hotels in Dublin, London, Hong Kong, Chicago, Las Vegas and Singapore.
When they analysed the opinions expressed by customers, some interesting patterns started to emerge.
“We saw that in hotels in Las Vegas, people were really interested in the elevators, or lifts. We’re not sure why, but we suspect it’s because people want to get to the casino floor quickly, or that because of the size of these hotels they could be waiting a long time for a lift to arrive.”
In Chicago, hotel guests seemed more interested in what they were walking on that they were waiting for, he adds.
“A lot of people were coming down hard on the cleanliness of the carpets. Who knows why – we are still learning a lot about these things – but we are latching on to these kinds of trends in the review content. It’s useful for hotels to know these patterns, and we are looking at how the information can be used to inform recommendations online.”
His lab also mined into consumer electronics reviews on a major online retail site, and found they could build an effective recommender system based on the user reviews alone.
“We didn’t need to rely on the star ratings or the purchasing information, the reviews themselves were enough, and they are out there, available freely online.”
Commercial opportunities
Smyth, who has already co-founded two companies based on his research at UCD (ChangingWords and HeyStaks), is now interested in working with industry to develop the ‘opinionated’ recommender approach further.
“We are always looking for commercialisation opportunities and we are wondering what would a start-up in this area look like – what sort or service offering would it have, what market would it focus on and would businesses want to buy into a toolset that we can provide.”
“This kind of analysis is possible today, and it could be commonplace in a few years.”
Fake reviews: can software smell a rat?
Mining opinion from user reviews might give you new perspectives on what consumers like and what they don't particularly warm to, but what if the reviews themselves are questionable? Is there a way to sniff out fake reviews online?
Prof Pádraig Cunningham (left), a director at The Insight Centre for Data Analytics, became interested in this area when he was checking out hotel reviews ahead of a holiday and noticed several overly positive testimonials on a hotel recommendation website. “I smelled a rat, and I thought the positive reviews had originated from the hotel,” says Cunningham, who is professor of knowledge and data engineering at UCD.
One of his research interests is detecting anomalous behaviour online, so he started to analyse online customer reviews and found that some patterns could hint that something wasn’t quite right. One of these patterns centres in the ‘singleton’ review, where a reviewer had made only one contribution, and it was overly complimentary.
“If you see a hotel that has a much higher proportion of singleton reviews than would be the norm, that can be a suspicious signal, particularly if they are positive reviews,” he says.
However, he notes that it’s not always clear cut. “Sometimes a hotel may be encouraging customers to leave a positive review if they had a good experience,” he says. “It’s referred to as ‘astroturfing’ because it creates an apparent grass-roots support, but these are actual customers, they are just being nudged.”
Cunningham’s group at UCD is now applying similar techniques to spot bullying behaviour online.
“From an algorithmic point of view spotting this is similar to the problem of spotting fake reviews,” he says.