The climate is changing, and we need to know what changes to expect and how soon to expect them. Earth system models, which simulate all relevant components of the Earth system, are the primary means of anticipating future changes of our climate.
Over the past century, our knowledge of Earth has advanced rapidly. Most research has focused on a single “sphere”, such as the atmosphere, the hydrosphere, the lithosphere or the biosphere. But all these spheres, and others, are connected, with strong interdependencies and feedbacks. For a comprehensive understanding, we need to take a holistic view: the climatology of our planet can be understood only if we treat Earth as a cohesive and integrated entity.
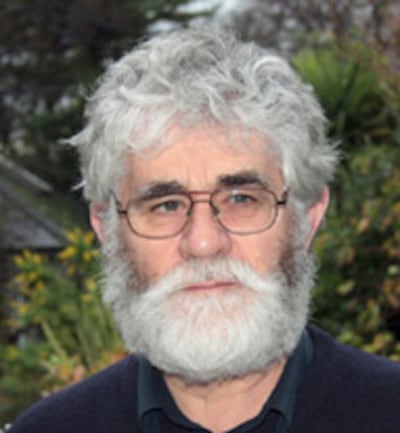
Earth system science brings together researchers from all the disciplines that study Earth. It combines meteorology, oceanography, geology, biology, ecology and geography and also embraces aspects of economics and sociology. Earth system science is crucial for understanding and tackling the problem of climate change. It provides a rational basis for studying the varying states of our planet in the past and enables us to anticipate imminent and more distant changes.
Biological processes are known to have had a powerful influence on the Earth system. The emergence of life strongly modified the chemical balances in the ocean and produced an atmosphere favourable for the development of intelligent life. But human influence on the atmosphere and oceans is now known to be significant and we need to find ways to minimise or avoid any further damage.
Revolutionised
The urgency of understanding the influence of the changing carbon cycle on climate triggered the development of Earth system models, which have revolutionised climate science. These models have evolved through a hierarchy, from simple one-dimensional energy balance models, through atmosphere-only models and coupled atmosphere-ocean models – global climate models or GCMs – to Earth system models or ESMs.
These models include representation of the global carbon cycle, dynamic vegetation, atmospheric chemistry, ocean biogeochemistry, sea ice and continental ice sheets. These models are able to represent human influence on climate due to deforestation and greenhouse gases emissions. ESMs can thus help us to gauge the effects of human policies, decisions and actions on the climate.
Climate predictions continue to be dogged by substantial uncertainties. The treatment of clouds is a particular source of problems
By emitting greenhouse gases, we are engaged in an uncontrolled experiment on Earth. An ESM provides a virtual planet on which we can perform controlled experiments – impossible on the real Earth. For example, we can double the atmospheric carbon content and generate the resulting climate. ESMs serve as laboratories, enabling us to study scenarios and estimate regional and global climate impacts under a wide variety of conditions.
Climate predictions continue to be dogged by substantial uncertainties. The treatment of clouds is a particular source of problems. Quantities are evaluated on a discrete spatial grid and scales smaller than the grid cannot be properly represented, although they may be essential for accurate predictions. The representation of biological and ecological processes is often crude, as governing equations for these are generally unknown.
Storm tracks
Weather prediction models have improved dramatically in recent decades. Climate models have undergone parallel improvements, with better simulation of El Niño and storm tracks. But climate projections still have unacceptably large uncertainty. For example, for the critical question of how much additional carbon will produce a warming of one degree, different models give widely differing answers. This makes planning very difficult.
There is hope that Earth system models can be radically improved by combining them with machine learning schemes. With emerging artificial intelligence techniques, models can learn and improve using observations and targeted high-resolution simulations of specific phenomena.